Reviewing Menlo Ventures’ Take on AI Agents and Why a New Architecture is Essential for 80%+ Automation
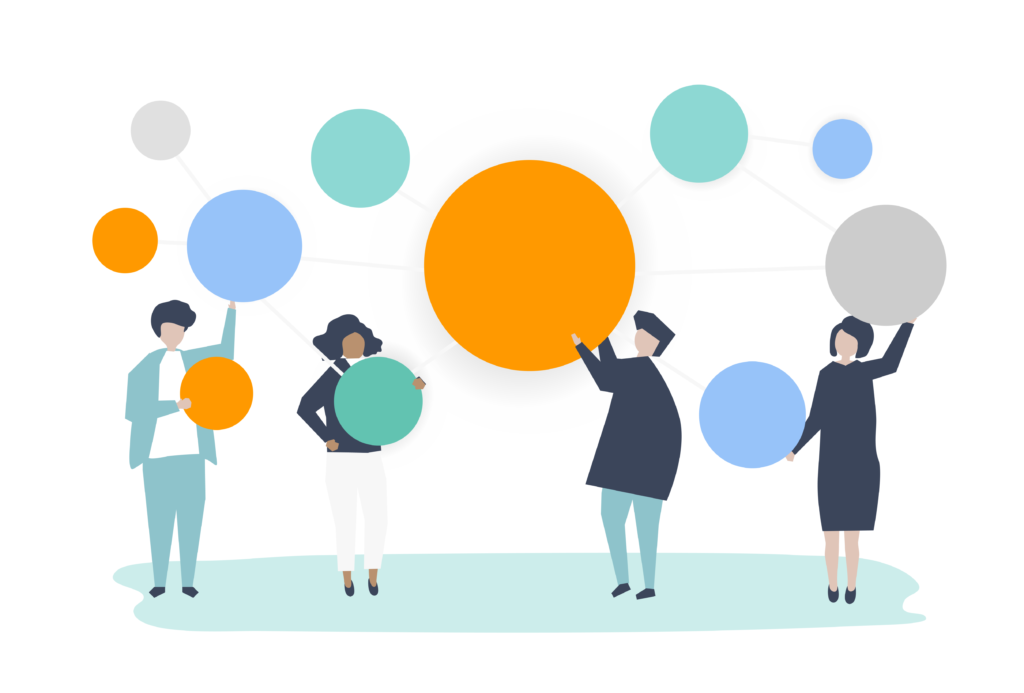
Home Thought Graph Resources Chrome Extension Contact Us Humberger Toggle Menu Reviewing Menlo Ventures’ Take on AI Agents and Why a New Architecture is Essential for 80%+ Automation In their recent article, Menlo Ventures beautifully outlines the evolving landscape of enterprise automation, focusing on the critical role that AI agents are now playing. Their piece […]
Lexie’s Thought Graph: A Jet Engine for Automation
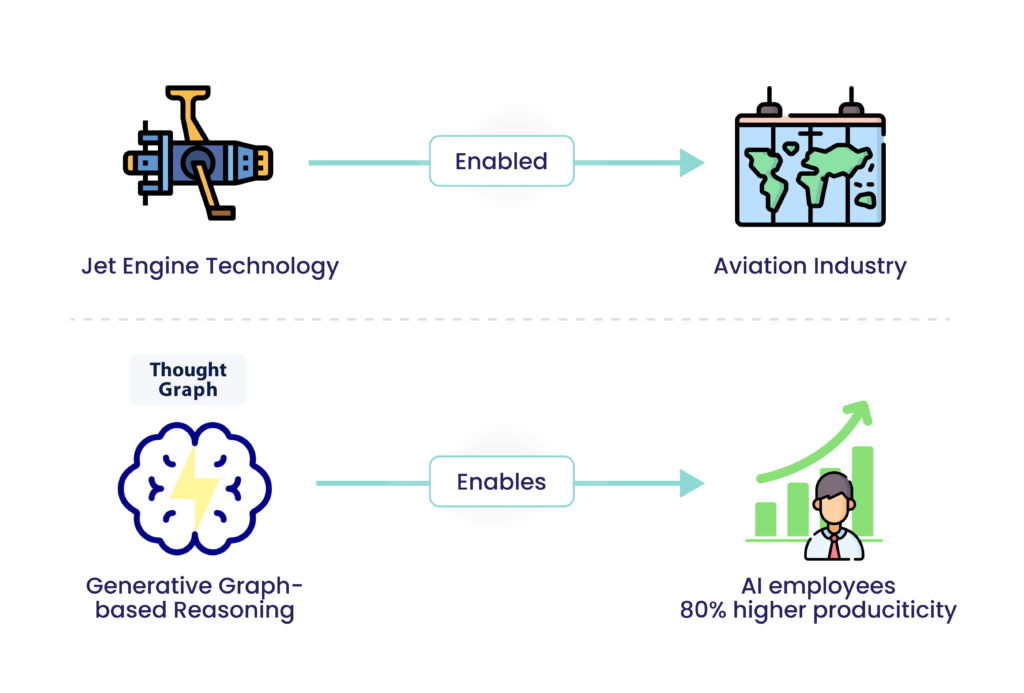
Just as jet engines democratized air travel, Lexie’s thought graph aims to make advanced automation accessible to everyone—from citizen developers to large enterprises.
AI SDRs Are Falling Short—Here’s How to Leverage AI to Transform Your Sales Organization
Home Thought Graph Resources Chrome Extension Contact Us Humberger Toggle Menu AI SDRs are Falling Short—Here’s How to Leverage AI to Transform Your Sales Organization Every day, it seems like a new AI sales development representative (SDR) company pops up. My colleagues and I often joke about how rare it is to go a day […]
Hello world!
Welcome to WordPress. This is your first post. Edit or delete it, then start writing!
Hello world!
Welcome to WordPress. This is your first post. Edit or delete it, then start writing!
Hello world!
Welcome to WordPress. This is your first post. Edit or delete it, then start writing!
Thought Graph and Visitor Workflow Model
Home Thought Graph Resources Chrome Extension Contact Us Humberger Toggle Menu Thought Graph and Visitor Workflow Model The AI transformation is a tangible reality now, and the pressing challenge is to democratize the power of AI for all, not exclusively for tech specialists. Over the last three years, our team at Lexie.ai has been dedicated […]
Natural Language Code Revolution – AI Leading the Way for Citizen Developers.
Home Thought Graph Resources Chrome Extension Contact Us Humberger Toggle Menu Natural Language Code Revolution – AI Leading the Way for Citizen Developers In 2017, Jensen Huan, CEO of NVIDIA, stirred up the tech world with his statement about “AI eating the software.” In recent years, AI code generation has gained significant traction, with OpenAI’s […]
Revolutionizing User Experience with AI: Chatbots, Co-Pilots, and AI-Native Apps
Home Thought Graph Resources Chrome Extension Contact Us Humberger Toggle Menu Revolutionizing User Experience with AI “Generative AI is just a phase. What’s next is interactive AI” said Mustafa Suleyman, the co-founder of Google’s DeepMind. Rich Miner, the founder of Android, at the AGI House event described the future of UX as follows: “We will […]
AI Reasoning: Balancing Generality and Reliability
Home Thought Graph Resources Chrome Extension Contact Us Humberger Toggle Menu AI Reasoning: Balancing Generality and Reliability In the ever-evolving landscape of artificial intelligence, the potential for transformative impact is monumental. According to Goldman Sachs, AI has the potential to exert a staggering $7 trillion influence on the global GDP. However, as AI rapidly advances, a new […]